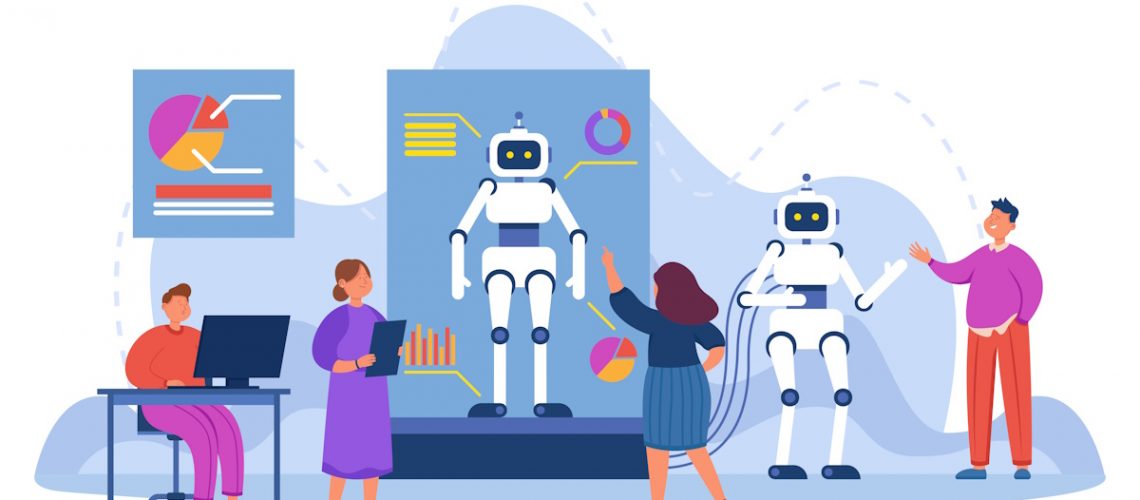
The Dangers Of AI Hallucinations To Automated Security Defences
AI hallucinations in cyber security: what do we know?
As organisations integrate more robust and innovative AI and automation tools into their business and security operations, the rising cyber threat landscape continues to evolve. Many cyber threats like phishing, ransomware, and distributed denial-of-service (DDoS) attacks are well known to security professionals, but new attack vectors and methods emerge seemingly by the day. Furthermore, alarmingly few people are aware of the threat of ‘hallucinations’ in large language models (LLMs) that are firmly intertwined with AI.
When we talk about AI hallucinations, we’re discussing AI-generated errors, false narratives, erroneous data, or falsified statistics, where a tool (such as the world-renowned ChatGPT, for example) produces plausible but inaccurate information. To the untrained eye, even if specific prompts were given to the AI tool, the dispensed output may seem convincing enough with no reason to suggest its validity. For every ethical argument that proposes using AI tools for content or image generation, it’s important to not overlook the inherent security risks present.
Where do AI hallucinations originate?
As AI hallucinations can exist in a plethora of tools, it begs the question of what would happen if misinformed or false data were to influence cyber security controls. Many cyber security and incident response tools themselves rely heavily on the autonomous and rapid gathering, aggregation, monitoring, and processing of data. Should there be inaccuracies or false positives generated by the LLM powering an AI tool, it could identify a benign or innocuous activity as a legitimate threat, or vice versa.
The way that LLMs are trained is the root cause of this underlying and persistent problem; they ingest massive datasets from the internet, but – if we’re to continue using ChatGPT as an example – that information is only valid for a certain period. ChatGPT is only trained on internet data up to April 2023, which, at this time of writing, could mean that information dispensed is fabricated, outdated or incorrect based on current events. A ChatGPT response may appear reasonable on the surface, but to another automated tool that lacks the innate ability to differentiate fact from fiction, it simply digests data verbatim.
As such, AI hallucinations can then lead automated security controls to believe threats are present when they aren’t, or that systems are safe despite a vulnerability being exploited. This has sparked concern, especially recently given that ChatGPT’s LLMs have been exploited by nation-state threat actors.
The consequences of AI hallucinations in cyber security
If an AI-powered, autonomous security solution were monitoring network traffic and encountered an unsuspecting false positive, the system may trigger disruptive and unnecessary countermeasures, including system lockdown, backup restoration and threat containment. Inherently speaking, algorithms cannot detect whether numerical or statistical data is falsified – they simply lack the innate ability to do so. Therefore, if the LLM powering the AI solution hallucinates incorrect data, it invariably forces the tool to react in ways that could waste valuable resources, lead to prolonged downtime, and increased disruption.
Conversely, the LLM may fail to recognise an authentic pressing threat and fail to alert the tool appropriately, thus leaving the network and connected systems vulnerable to compromise. This oversight could result in a broad spectrum of attacks with no evidence to suggest that suspicious activity has taken place.
Given cyber security professionals rely on automated data gathering to investigate, contain, and respond to security incidents, the risk of AI hallucinations is particularly worrying. If false data is accrued, inaccurate findings and reports are more likely, and ultimately the data that drove certain decisions must be questioned for its authenticity. As such, the presence of AI hallucinations potentially risks hampering a company’s ability to effectively isolate and mitigate cyber attacks, as well as the covert activity that leads to them.
If misinformed or false AI-generated data were to reach the public domain, whether in the form of an organisation divulging inaccurate statistics, or the fact that the organisation was revealed to have relied on skewed data to begin with, it could have disastrous reputational damage. Putting aside the varying opinions about AI-generated content, transparency must be a priority for every solution involving AI, meaning that organisations risk undermining trust and credibility among their customers, partners, and stakeholders.
Furthermore, malicious actors can leverage AI in highly sophisticated ways, such as by manufacturing code packages that appear legitimate but contain hidden malware. When users unknowingly install these files, they unwittingly expand the attack surface of systems and networks.
How to prevent and deal with AI hallucinations
To address the risks posed by AI hallucinations in cyber security functions and contexts, organisations must adopt a multi-faceted approach.
1. Enhance Validation Protocols
Cyber security professionals should establish robust and watertight validation protocols to ensure that AI-generated data and outputs are checked rigorously and regularly. This will likely involve the comparison of information against a multitude of sources to verify its accuracy and implement automated validation tools to detect anomalies and potential hallucinations. However, the fundamental basis for this validation step is to incorporate a stringent practise of human oversight and expert reviews.
2. Diversify and Expand Training Data
Outdated or incomplete training data is often the basis for false AI-generated information, and given how convincing such content may appear, it’s important to exercise caution and review the data that has led to that perception. Organisations should regularly update and review their AI models and integrations with up-to-date threat awareness/intelligence, security best practices and other relevant, credible information to ensure that the models are making decisions based on accurate data.
3. Establish Clear Policies and Guidelines
The use of AI tools in a cyber security operation should not be championed haphazardly or without clear policies and guidelines in place. Organisations should consider reviewing and enhancing their policies to define specific use cases where AI can be leveraged effectively, or mandate that employees complete a validation checklist before AI-powered data can be dispensed.
Fundamentally, AI cyber security policies serve as a reliable point of reference for escalation procedures in case AI data is deemed unreliable or suspicious, and the same can be applied to alerts that stem from false positives.
4. Foster Ethical Human-AI Collaboration
While AI tools can provide valuable assistance, cyber security professionals should not solely rely on them. By maintaining a collaborative approach, where AI and human experts work together, organisations can leverage the strengths of both to make more informed and reliable decisions.
As automation and AI in cyber security continue to grow, firms must be vigilant in addressing the risks posed by AI hallucinations. The steps above will help organisations build more resilient and reliable defences, while fostering a collaborative and transparent culture around cyber security will ensure these prevention methods are deployed effectively.
To ensure your security defenses are equipped to handle the challenges posed by AI hallucinations, consider Phishing Tackle’s cybersecurity awareness training and real-world simulated phishing resilience testing. Our comprehensive solutions provide you with all the tools and strategies needed to identify and address vulnerabilities before they can be exploited. Book a demo today to see how it can work for you.